This blog is the fifth of a series on AI business challenges, written by Xomnia’s analytics translators.
Being data-driven is becoming the norm for organizations of all sizes and in all industries, as they continue to explore the opportunities that data and AI have to offer.
In earlier blog posts, we dived in-depth to explore some important topics that are tailored to help organizations in determining and achieving their data-driven goals. Such topics included setting a strong data and AI strategy, defining and starting data products and proving the value of data projects.
In this blog, we take a step further and look at running projects that are not delivering value and seem stuck. This includes data projects that are struggling from a decrease in the development pace, team motivation and/or stakeholder involvement - as well as other possible scenarios. Executives and product managers will find it particularly interesting to diagnose these issues and find a solution to get the project back on track.
How analytics translators can help in handling strained data projects
Data and AI are relatively new domains for almost every organization. They require new expertise, infrastructure, and roles. Especially in the case of AI, a new paradigm on how to deal with the uncertain outcomes of the effort is necessary (see our whitepaper). As analytics translators, our role is to give advice to organizations that find it difficult to align all these elements and run successful projects. For this purpose, we have developed a project diagnostics approach, which helps smoothen projects, get them back on track and make them excel. Our approach, which you can dive into by downloading our way of working whitepaper, starts with understanding the current situation, which allows analytics translators to dive into the project and diagnose the issues.
Identify the issues that withhold project success involves multiple methodologies. At Xomnia, we identified three main focus areas and their underlying issues to determine why a project is strained:
- The 'Why': addresses the reason a product is not delivering its promised value
- The 'What': addresses the product itself, and whether or not it is the right one
- The 'How': addresses whether the required data capabilities for the data project are lacking
As analytics translators, we present the identified issues and their solutions in an actionable plan. After receiving the green light of the stakeholders, the plan is executed to bring the project back on track. In parallel, we present best practices and share them with other teams.
In this blog, we will take a closer look at the main issues and their corresponding fixes.
An issue with the ‘Why’ of a data product: Product is not delivering its promised value
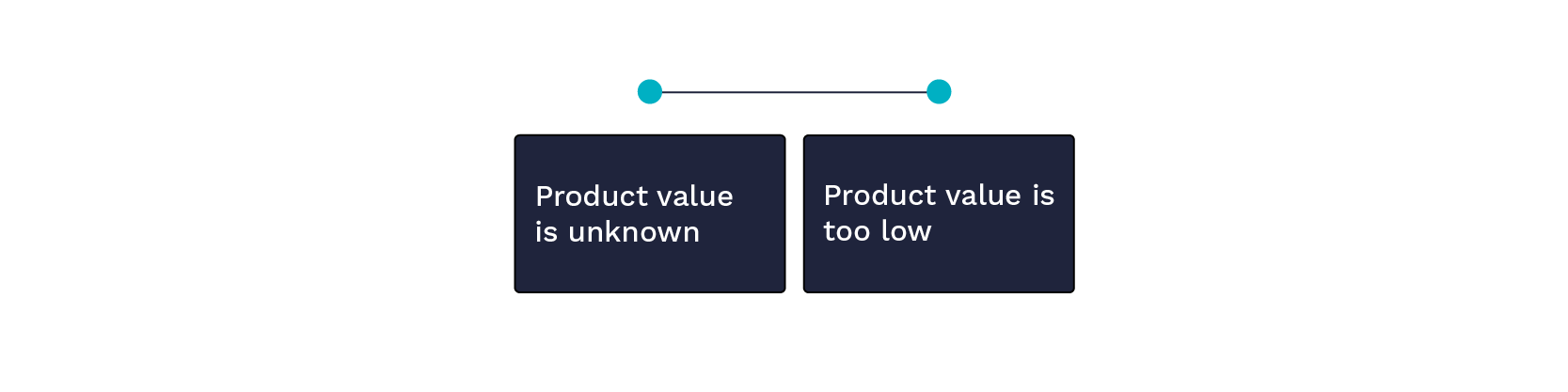
To determine if a data product is living up to its promise, you need to know 1) what this promise entails (what are the calculated business benefits?), and 2) how the product is performing (which of the predefined business benefits are being achieved?). When a data product’s business case is not defined, it’s hard to prove the success of the project to stakeholders. You can then either choose to reestablish a product’s raison d’être (it's 'why') or move on to actually measuring its impact.
One way to measure the value of the project is to deploy small pieces of work as fast as possible in the form of a minimum viable product (MVP). The MVP can then be tested and evaluated with the end-users and stakeholders, who then share the value in a qualitative (e.g., improved decision-making capability) or quantitative (e.g., decrease in production costs) manner.
When the product’s value is too low, start investigating with relevant stakeholders the reason behind that. Next, use the outcome of this investigation as input for brainstorming sessions about value-adding features with the end-users, SMEs and other stakeholders.
If there are insufficient features to generate value, consider ‘killing your darling’: abandoning the project and accepting the loss.
Need more help? Do not hesitate to contact Xomnia's data experts
An issue with the ‘What’ of your data product: The wrong product is being developed
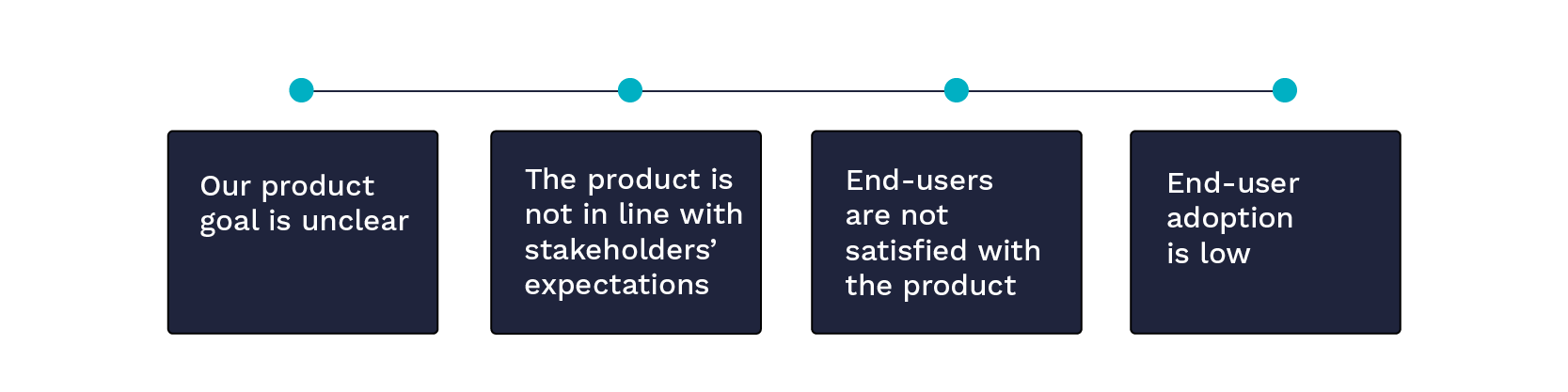
One of the primary challenges in the face of a data project is the lack of clarity about what you want to develop. A good way of checking if you’re developing the right data product is to ask yourself a few questions:
- Is the data product goal clearly defined?
To answer this question, the ‘why’ of the data product should be clear, and it mainly results from the organization-wide data strategy. Start (re)investigating the strategic purpose of the data product with stakeholders and end-users, and (re)defining a data product goal together that reflects the scope of the project.
Xomnia's use case canvas can be a good starting point for the data product goal development process. Keep thinking critically about whether the data product goal is adhered to during the development process or not, thus avoiding wasting time on unnecessary tasks. If needed, change the data product goal during the development process so that it is in line with the data strategy and the needs of the stakeholders and end-users.
2. Does the data product fulfill stakeholders’ needs?
To know whether the data product fulfills stakeholders’ needs, you first need to identify all stakeholders. Creating and updating a stakeholder matrix together with subject matter experts will help in identifying the stakeholders. Identify and refine the expectations of the stakeholders throughout the product development process and conduct regular meetings to review the current work and share the outlook for the coming period. By doing so, the product features will align with the stakeholder's expectations, and will ensure that impediments are identified as early as possible.
3. Are end-users satisfied with the data product’s results?
To identify whether end-users are satisfied with the data product, all of those end-users need to be identified and involved in the product development process as early on as possible. For instance, integrate end-user feedback in your development process by applying agile principles. In addition, maintain close contact with a super user, who can be the voice of all the users, to identify how satisfied end users are with the data product.
4. Are users adopting the data product?
Product adoption is crucial to prove the value of a data product and to sustain it in the organization. Before knowing if users are adopting the data product, monitoring product usage needs to be in place, for example, by tracking the number of user interactions with the data product.
User adoption can be low because end-users are not involved early on in the development process. This can result in data products that are too complex for the end-user, not aligned with the business processes or wrongly used. Due to all those reasons, it’s key to involve end-users early on in the development process, this can be done through end-user workshops, where they can share their thoughts and experiences. A transformation plan which includes training, super users, product reviews, etc. can also help in increasing the user adoption of a data product.
To conclude, it is critical to (re)define a realistic data product goal that is consistent with the company's overall strategy. When (re)defining the data product goal, the first step is to involve stakeholders, end-users and subject matter experts. Product adoption will be low if end-users do not recognize the added value of the product. Subject matter experts will assist in the (re)definition of a product goal so that it is aligned with the business processes. Equally important when (re)defining your data product goal is identifying (key)stakeholders and their expectations. It is vital to involve stakeholders in the project as early as possible to avoid organizational resistance during the project.
Xomnia has created several tools (e.g., use case canvas, stakeholder matrix, end-user workshops, etc.) to help accelerate and streamline the process of (re)defining the right data product goal. To know more about those tools, do not hesitate to get in touch.
An issue with the ‘How’: Required data capabilities for the data project are lacking
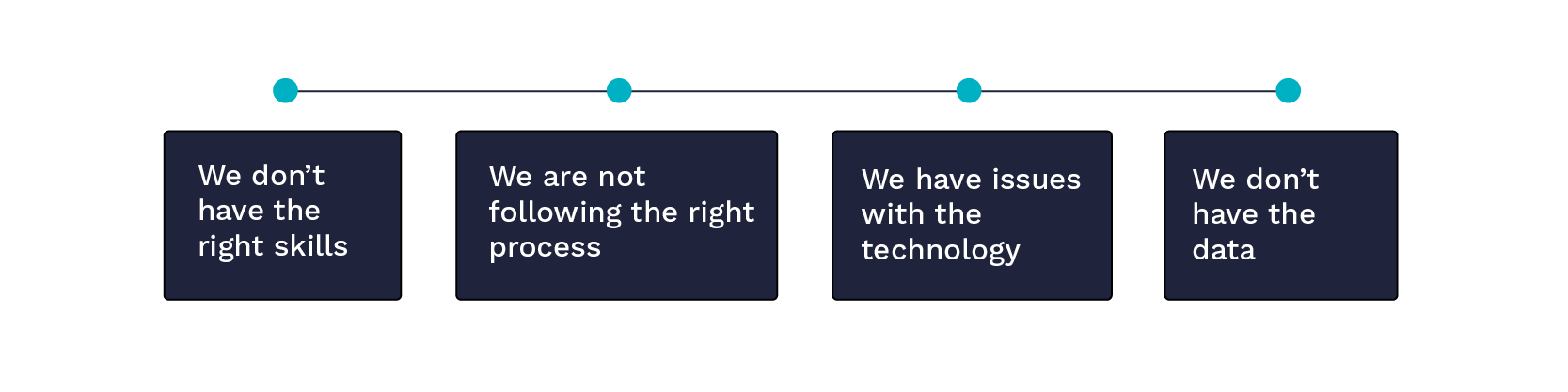
You know you’re developing the right data product - but are you developing that data product right? Not having the right skills for the project is one of the main issues of difficult data projects. Therefore, it is important to (re)define which expertise is needed for the project is crucial for the project to succeed. Each phase of the project may require different expertise, and by continuously (re)thinking about this, the chances of strain are reduced. Over time, the involvement and motivation of internal team members and stakeholders within the organization can decrease. Therefore, continuously making sure that everyone is dedicated to the project prevents the project from becoming strained. Selecting and applying the right project management approach (e.g., agile, scrum, Kanban, Lean) helps in keeping the involvement and speed high during the project.
In addition to having the necessary expertise, the right infrastructure and tools to quickly extract and analyze data are critical to the project's success. Therefore, it is important to consult with solution architects about which tools and infrastructure (e.g., data platform) are required when and where in the project. It is important to take current and future project data needs into account when selecting tools and setting up or adjusting the data platform.
At Xomnia, we have built a streamlined approach on how to set up and adjust the data platform. Overseeing all data governance-related topics (e.g., compliance, legal, privacy, data quality) when (re)building a data platform can help reduce the risk of a project becoming strained. Allocate sufficient resources to the technical backend and strive to automate as much as possible throughout the project to avoid error-prone and time-consuming manual labor.
Last but not least, another significant constraint for a data project is a lack of appropriate data. When it turns out that the data for the project is not available, start collecting the data in different ways (e.g., sensors, questionnaires) or look for alternative data sources (e.g., synthetic data). Issues regarding the extraction of data can strain the project development; therefore, a solid data platform and data engineering expertise are important for the project to succeed.
Get in touch with our team of analytics translators
—-
Xomnia has a team of analytics translators (ATs) dedicated to assisting clients with the business aspects of AI challenges. They do so in several roles, such as AI Product Owner (product-focused), AI evangelist (adoption-focused), AI ethicist (responsibility-focused), or Strategic AI advisor (strategy-focused). In this last role, our ATs tackle the first of seven AI business problems (creating a strong AI vision and plan aligned with the overall business strategy - topic of this blog). In our next AT blog, we’ll discuss our proof of value method.
Would you like to learn more or have a conversation about AI business challenges at your organization? Get in touch: info@xomnia.com.