AI is an integral part of today's society, offering the possibility of smart solutions to improve it. We experience AI's benefits on a daily basis ourselves, where algorithms are used to make our lives easier (think smart heating systems and autonomous driving and sailing), more efficient (think recommendations made by your search engine or favorite streaming service), healthier (think AI applications to combat pollution), safer (think fraud detection applications), and the list goes on.
On the other hand, there are also disadvantages/challenges to using AI. Those, unfortunately, can be demonstrated by a myriad of examples of data breaches. A world-famous example of conscious data and AI misuse include the illegal sharing of data of around 87 million Facebook users with the dubious data consulting firm Cambridge Analytica.
Data and AI misuse can be also unconscious, due to issues related to biases, fairness and representativeness. For example, COMPAS is an algorithm used in US court systems to predict the likelihood that a defendant would become a recidivist. Due to the data that was used, the model that was chosen, and the process of creating the algorithm overall, the model predicted twice as many false positives for recidivism for black offenders (45%) than white offenders (23%)*.
As misuses and breaches of data are increasingly coming to light, the protection of personal data is more topical than ever. Customers nowadays demand guarantees to have control over their personal data, and that this data is safeguarded well. Only then will they be happy to share their data, which can be used in turn to improve your service via data science and AI solutions.
The introduction of the GDPR (General Data Protection Regulation), and more recently the EU Artificial Intelligence Act, plays a major role in bringing incidents of data & AI misuse as well as data breaches to light, by obliging companies to report incidents.
Don't miss Xomnia's events about the latest trends and news in the world of AI. Click here.
What is the data protection act (GDPR) and what does it cover?
The mentioned challenges for - and incidents of - data misuse emphasize the importance of rules that regulate how companies deal with personal data. Most companies collect a large amount of data, which should be handled responsibly.
The GDPR stands for General Data Protection Regulation, which is a law that has been valid since May 2018. It is a stricter replacement of the EU’s Data Protection Directive, which went into effect in 1995, well before Social Media and e-commerce became as dominant as they are today.
GDPR primarily aims to give individuals in the EU control over their personal data, by regulating the way their privacy-sensitive data is processed. Privacy-sensitive data is information that can be traceable to a specific person, such as the citizen service number, address, date of birth… etc.
The philosophy behind GDPR is that customers should be able to trust organizations to keep their data. In order to do so, the European Commission has formulated 7 principles of the GDPR:
What are the 7 principles of GDPR?
1) Lawfulness, fairness and transparency: Obtain the data on a lawful basis; inform the person concerned fully and stick to the agreements.
2) Purpose limitation: Personal data is collected for a specified legitimate purpose and may not be used for any other purpose.
3) Data minimization: Only the necessary data required for the intended purpose should be collected.
4) Accuracy: Store accurate, up-to-date data.
5) Storage limitations: Personal data must not be stored longer than necessary for the intended purpose.
6) Integrity and confidentiality: Personal data must be protected from unauthorized access, loss or destruction.
7) Accountability: Record and prove compliance. Ensure policies are followed.
Don't miss Xomnia's latest business cases and blogs. Subscribe to our newsletter.
How to take the right measures to become GDPR compliant?
Work on your data organization
Organizing all the data processing and predictive modeling activities within your organization to become GDPR compliant could pose a challenge, especially when these kinds of activities take place in a decentralized manner within different departments or business units.
It’s not unusual that your new development and production environments on analytics looks like the IT spaghetti of the past. However, this messy environment is not sustainable in GDPR terms.
At Xomnia, we advise many customers to move away from “stitched together” environments to controlled environments, in which all users have access with the proper access controls, and all their actions are traced from the source to the predictive model. Both data and predictive models are version controlled.
Have a data inventory
When it comes to the GDPR, organizations will need to take stock of where all the data is stored and ensure that it is accessible, but only to those who have the proper mandate to do so.
Managers of analytical teams should be able to easily understand and audit data sources, while being able to answer questions like 'who has access to what?' and 'what sources are being used for which projects?'. The same goes for Data Protection Officers (if applicable) under the GDPR.
Have reproducible results
GDPR holds firms accountable for bias and discrimination in automated decisions. Moreover, companies may not use specific categories of personal data in automated decisions, except under defined circumstances. Therefore, in organizations that use automated decision-making, GDPR creates a “right to an explanation” for consumers.
Prevent data leakages
One of the requirements of the GDPR is that, by using appropriate technical and organizational measures, personal data must be processed in a manner that ensures the appropriate security of the personal data. This includes protection against unauthorized or unlawful processing and against accidental loss, destruction or damage.
Depersonalize data
As engineers and scientists, we make sure that we never receive personal data in the first place. We ask customers for pseudonymized data, so that they aren’t at risk of breaking the law. It’s important to think about safeguarding privacy with all of the parties involved. It’s a shared responsibility, and companies should involve their privacy officer to make sure that protocol is being followed.
Protect your data by figuring out which information is traceable and what you can do to make it non-traceable. Personal data isn’t vital to complete an analysis, and using depersonalization means you can avoid breaking the law when you analyze the data, or if you share it with a 3rd party for analysis.
Aggregation is a technique to depersonalize data by categorizing data. Categorizing is a way of creating pseudonyms for the data so it’s depersonalized. You can do this by putting age as a category, or postcodes only including letters, for example. You could also make your data anonymous, but then you won’t be able to link the data, which isn’t ideal for analysis.
Investigate risk level of your AI product according to AI Act
Always keep the AI Act in mind when developing an AI Solution. Investigate which risk level is applicable to your product, and develop it according to the corresponding regulations.
Join our team of top-talent data and AI professionals. Click here to view our vacancies.
What does the newly proposed EU Artificial Intelligence Act add to existing GDPR law?
Besides the existing GDPR, The European Commission came up with a new proposal specifically aimed at AI: the Artificial Intelligence Act. This proposal aims to guarantee the safety of AI products within the European market, and to make Europe fit for the digital age, by enhancing the trust in - and sequentially boosting the usage of - AI solutions within society.
It is important to note that the AI act is still a proposal that might need at least another 2 years to pass through the European Council and European Parliament and become fully effective.
Even though the proposal is bound to change from the way it is currently defined, it shows an intent to regulate AI and the risks it entails for users, data subjects, personal rights, and societal values. As of now, the framework of the EU Artificial Intelligence Act is a risk-based approach to regulation:
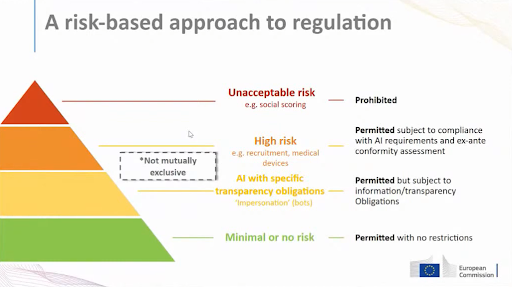
As can be seen from the picture, only a small part of the AI solutions is labeled as unacceptable risk, and thereby prohibited under the EU AI regulation. There are also AI solutions labeled as high to unacceptable risk and are hence subject to regulations due to the EU AI Act. These solutions are not prohibited but need to be compliant with the requirements as formulated by the EU AI Act. The majority of AI solutions have no obligations with regard to the EU AI Act, even though transparency is highly recommended.
Therefore, the majority of AI solutions will not be affected under the AI Act, as it mainly increases the awareness around the development of AI products and the impact on human rights. More detailed information on the EU AI Act can be found on:
What is the impact of the GDPR & AI Act on artificial intelligence and data science?
The GDPR, and potentially the EU AI Act, do not make data science impossible. They are designed to be regulations that help clarify the right measures to increasing the safety and trust when using data and AI, and hence make data science and AI solutions possible.
*Refer to ProPublica publication. It's worth mentioning that the rate of false negatives was also skewed: the false negative rate for white offenders is 48%, whereas the rate of false negatives among black defendants is 28%. So, in comparison to black people, white people are not only more likely to be misclassified as non-recidivist, but they are also less likely to be misclassified as recidivist.
Achieve your organization's full potential with the leading AI consultancy in the Netherlands